Why JuiceFS?
Tens of billions of files
As the parameter sizes of large language models and other foundation models grow, their training datasets expand significantly. JuiceFS can manage up to tens of billions of files in a single volume. This capability has been proven in multiple enterprises' production environments, making it ideal for large-scale AI datasets.
High aggregate throughput
With flexible cache configurations, JuiceFS provides virtually unlimited aggregate throughput. By leveraging multi-level cache strategies, priority-based eviction policies, and capacity weighting, JuiceFS maximizes existing hardware resources and eliminates the need for additional dedicated hardware investments.
Efficient large file writes
Checkpoint saving in large language model training involves extensive large file writing. JuiceFS uses a block storage design, combined with enhanced concurrency for object storage access and write caching, to optimize sequential write throughput for large files. This effectively reduces GPU idle time.
Cloud-native design
Designed specifically for cloud environments, JuiceFS can be deployed on global public clouds and seamlessly integrates into existing cloud infrastructures. This meets diverse platform and regional requirements.
Multi-cloud file systems
When GPU resources are distributed across regions, ensuring on-demand remote data access and addressing bandwidth limitations is critical. JuiceFS' mirror file system ensures consistent and localized data access worldwide. Data replication cost is lower than bandwidth cost, reducing cross-region access expenses and optimizing data distribution.
Cost-effective architecture
JuiceFS' architecture separates performance and capacity: it leverages cloud-based, highly available, elastic, reliable, and cost-effective object storage for capacity; it uses NVMe SSDs near compute nodes as cache to ensure high-performance access. This transparent cache mechanism offers you a seamless, efficient experience.
Feature Overview
MiniMax Built a Cost-Effective, High-Performance AI Platform with JuiceFS
MiniMax, a leading general AI technology company, adopts a hybrid cloud strategy to balance flexibility and cost efficiency. With GPU resources deployed across both IDC and cloud environments, JuiceFS provides a unified data access experience. MiniMax selected JuiceFS Enterprise Edition as the storage solution for its AI platform to ensure high-performance data access for various scenarios, including data cleaning, model training, and inference. [Learn more]
BentoML Reduced LLM Loading Time from 20+ to a Few Minutes with JuiceFS
BentoML, an open-source framework for large language model (LLM) AI applications, faced slow cold starts when deploying models in a serverless environment. Due to their large size, LLMs had long startup times, and limited image registry bandwidth worsened the issue. To solve this, BentoML implemented JuiceFS. As a result, BentoML reduced model loading times from over 20 minutes to just a few minutes.. [Learn more]
Trusted by Innovators in Gen-AI
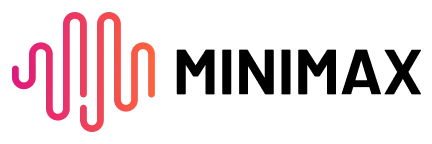
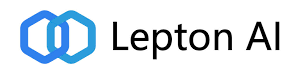
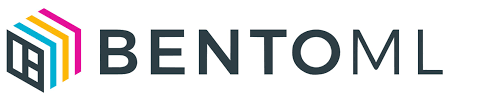
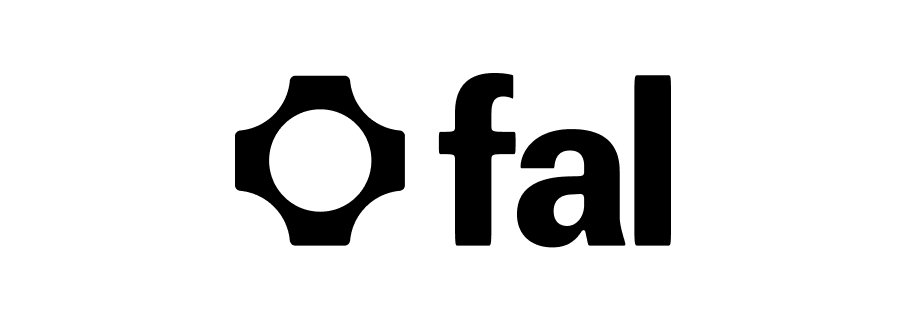
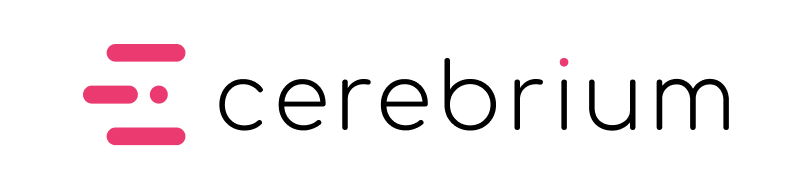
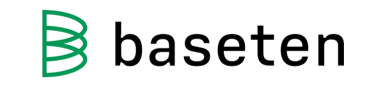
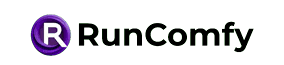
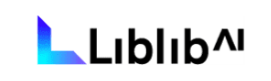